What is the Significance of This Novel Concept? A Comprehensive Overview of a Groundbreaking Approach.
This approach, a key component of modern analysis, leverages a unique methodological framework. It encompasses a structured system for investigating complex phenomena, offering detailed insights and predictive capabilities. Examples of its application are evident across a broad range of fields, including but not limited to [mention a field, e.g., data science or financial modeling]. Its efficacy stems from a rigorous combination of data collection, statistical modeling, and strategic interpretation. This framework is particularly valuable in identifying patterns, relationships, and causal factors, ultimately assisting in decision-making and problem-solving in a variety of complex situations.
The importance of this approach lies in its potential to revolutionize problem-solving in numerous disciplines. By allowing for in-depth analysis and predictive modeling, it offers a significant advantage over traditional methods. Its benefits extend to improved forecasting accuracy, more efficient resource allocation, and a deeper understanding of complex systems. The historical context of this concept is one of continual evolution, spurred by advancements in computing power, data availability, and statistical modeling techniques. This dynamic evolution underscores its enduring relevance and adaptability to future challenges.
Category | Details |
---|---|
Date of Birth | Not Applicable - Concept, not a person |
Field of Expertise | Complex systems analysis, predictive modeling |
Significant Accomplishments | Enabling innovative solutions in [mention specific domains or industries] |
Moving forward, this article will delve into the specific applications and practical implications of this innovative approach. Different real-world scenarios, practical examples, and detailed case studies will demonstrate its effectiveness and utility. This detailed exploration will provide valuable insights and understanding of its wide-ranging implications.
eorme
Understanding the multifaceted nature of "eorme" requires examining its core components. This exploration highlights key aspects crucial for a comprehensive grasp of this concept.
- Data Collection
- Pattern Recognition
- Statistical Analysis
- Predictive Modeling
- Problem Solving
- Decision Support
- System Understanding
These seven aspects, interwoven, form a robust framework for investigating complex issues. Data collection forms the foundation, leading to pattern recognition and statistical analysis. This data-driven approach enables predictive modeling, facilitating better problem-solving and informed decision-making. Effective system understanding arises from this process, offering a clearer picture of interconnected relationships and contributing to more effective solutions. For instance, in financial modeling, data collection on market trends, combined with pattern recognition and statistical analysis, leads to more accurate predictive models, supporting better investment decisions.
1. Data Collection
Effective data collection is fundamental to the "eorme" approach. The quality and comprehensiveness of data directly influence the accuracy and reliability of subsequent analyses. A robust methodology for gathering data is crucial for extracting meaningful insights and achieving desired outcomes. Without meticulous data collection, the entire framework of "eorme" becomes significantly weakened.
- Data Source Identification and Selection
Precise identification of relevant data sources is essential. This involves determining the most appropriate repositories, databases, and information channels. Carefully selecting the right data sources ensures that gathered information accurately reflects the phenomenon under investigation. For example, a study on consumer behavior might leverage sales data, website analytics, and social media interactions. The appropriate choice of sources directly impacts the validity of conclusions drawn using the "eorme" framework.
- Data Integrity and Quality Control
Ensuring data integrity is paramount. This includes meticulous validation of data accuracy, consistency, and completeness. Addressing potential biases and inconsistencies in the data is essential. For instance, in financial modeling, inaccurate transaction data will lead to unreliable predictions and flawed decisions. The quality control measures implemented at this stage directly impact the reliability of conclusions drawn using the "eorme" approach.
- Data Structuring and Standardization
Data needs to be formatted and organized to facilitate analysis. Standardizing data formats enables efficient processing and comparison. The structuring ensures compatibility across different datasets and minimizes potential errors during the analysis process. For example, a large dataset about customer preferences must be structured in a standardized way to ensure consistent interpretation.
- Data Volume and Frequency Considerations
The volume and frequency of data collection significantly impact the depth and breadth of insights. Sufficient data volume is needed for accurate representation of the phenomenon under study. The frequency of collection determines the timeliness of analysis and predictive capabilities. For instance, tracking stock prices over a longer period (high frequency data) provides more accurate predictions compared to less frequent data collection.
In summary, meticulous data collection, encompassing appropriate source selection, data integrity measures, standardization, and appropriate volume and frequency, forms a critical foundation for the efficacy of the "eorme" process. The quality of the data directly impacts the accuracy and usefulness of the insights generated, highlighting the paramount importance of this initial stage in the broader "eorme" framework.
2. Pattern Recognition
Pattern recognition is a fundamental component of the "eorme" framework. The ability to identify recurring patterns within data is crucial for extracting meaningful insights and developing predictive models. This process allows for the discovery of relationships, trends, and anomalies that might otherwise remain hidden. Successful pattern recognition hinges on the quality and volume of data available. Sophisticated algorithms are employed to process and analyze this data, revealing potential patterns obscured by sheer volume or complexity.
The practical significance of pattern recognition within "eorme" is evident in diverse fields. In financial markets, identifying recurring price patterns aids in forecasting potential market movements. In medical research, recognizing patterns in patient data can lead to early diagnoses and the development of targeted treatments. Similarly, in cybersecurity, identifying patterns in network traffic can help detect and prevent malicious activity. These examples illustrate how recognizing patterns can lead to improved decision-making and more effective problem-solving strategies. For instance, in customer relationship management, identifying patterns in customer purchase history allows businesses to tailor products, services, and marketing strategies, leading to increased customer satisfaction and loyalty.
In conclusion, pattern recognition is not merely a step within "eorme," but a crucial element driving its effectiveness. Its ability to reveal hidden relationships and trends makes it an essential tool for predicting future outcomes, optimizing processes, and addressing complex challenges across numerous domains. The challenge lies not in the availability of algorithms but in the quality and volume of data used for analysis. Accurate and comprehensive data sets are critical to achieving reliable and meaningful pattern recognition results, ultimately enhancing the efficacy of the "eorme" process.
3. Statistical Analysis
Statistical analysis is integral to the "eorme" framework. It provides a rigorous method for interpreting the patterns identified in collected data. Without statistical analysis, the potential insights embedded within the data remain obscured. Statistical techniques quantify relationships, assess significance, and generate reliable predictions. These quantitative measures provide a firmer foundation for decision-making compared to purely qualitative observations. For example, in financial modeling, statistical analysis of market trends allows for quantifying the probability of price movements, enabling more informed investment strategies.
The importance of statistical analysis stems from its ability to evaluate the validity and reliability of patterns. It determines if observed patterns are due to chance or represent genuine underlying relationships. Statistical measures such as correlation coefficients, hypothesis tests, and regression models provide objective evidence to support or refute claims made from observed patterns. In medical research, statistical analysis allows researchers to assess the effectiveness of treatments by comparing results across different treatment groups, thereby quantifying the impact and establishing causality. Without statistical analysis, the interpretation of patterns in medical data would be inherently subjective and less reliable for drawing conclusions about treatment efficacy.
In summary, statistical analysis within the "eorme" framework plays a critical role in validating patterns and drawing meaningful conclusions. The rigorous quantitative approach enhances the reliability of insights and contributes to more effective decision-making. Challenges may arise from data limitations or the complexity of the phenomena being studied, but the inherent rigor and objectivity of statistical analysis are paramount for mitigating these concerns and ensuring robust findings. This analytical approach enhances the overall validity and practicality of conclusions derived through the "eorme" methodology.
4. Predictive Modeling
Predictive modeling, a core element of the "eorme" framework, leverages patterns and statistical insights to forecast future outcomes. Its application within "eorme" hinges on the accuracy and reliability of the underlying data and analysis. The strength of predictive modeling lies in its ability to anticipate future trends, enabling proactive responses and informed decision-making.
- Data-Driven Forecasting
Predictive modeling in "eorme" relies on historical data to establish patterns and relationships. By analyzing past occurrences, models can project potential future scenarios. For example, analyzing sales data over several years allows for the prediction of future demand, guiding inventory management and production strategies. A model trained on historical weather data can predict future precipitation patterns, assisting in agricultural planning or disaster preparedness.
- Model Selection and Validation
Choosing the appropriate model is crucial. Models vary in their complexity and the types of data they handle effectively. Linear regression might suffice for straightforward relationships, while more sophisticated algorithms are required for complex systems. Validation through rigorous testing against independent data sets is essential to ascertain a model's reliability and its capacity to generalize to new situations. This ensures predictions are not overly influenced by the specific data used for model training.
- Parameter Tuning and Optimization
Model performance depends on the parameters used. Tuning these parameters can significantly impact prediction accuracy. Optimization techniques are used to fine-tune model parameters, ensuring optimal performance and minimizing prediction errors. This ensures that the model aligns closely with the observed data patterns. In financial modeling, optimizing parameters of a model predicting stock prices can enhance the accuracy of forecasts and inform investment strategies.
- Scenario Analysis and Sensitivity Testing
Predictive models often enable scenario analysis. By testing different potential scenarios, the model reveals how predicted outcomes may vary based on different circumstances. This provides a nuanced view of the future, allowing for adaptation and contingency planning. A business using predictive models for demand forecasting could test different economic scenarios to adjust production and inventory plans accordingly.
In conclusion, predictive modeling within the "eorme" framework plays a crucial role in extracting actionable insights. By forecasting future trends based on historical data, predictive models facilitate proactive decision-making and resource allocation. The success of these models depends on diligent data handling, model selection, parameter optimization, and scenario analysis. This process generates predictions that provide a structured basis for anticipating potential outcomes and mitigating potential risks in complex situations.
5. Problem Solving
Problem-solving is a crucial application of the "eorme" framework. Effective problem-solving depends on a structured approach that leverages the insights gained from data collection, pattern recognition, statistical analysis, and predictive modeling. This structured methodology provides a framework for identifying, analyzing, and resolving complex issues, offering a significant advantage over intuitive or haphazard approaches.
- Defining the Problem Clearly
The process begins with a precise definition of the issue. This involves identifying the root cause, scope, and potential impact. Vague or incomplete problem definitions can lead to ineffective solutions. For instance, a manufacturing company experiencing declining sales needs a precise definition of the problem is it a lack of marketing, a supply chain issue, or a drop in consumer demand? An accurate problem statement is the foundation for a targeted solution within the "eorme" context.
- Data-Driven Analysis
Thorough analysis of relevant data is essential. This involves gathering information from various sources, examining patterns, and utilizing statistical tools. An analysis of sales figures, customer feedback, and market trends provides a data-driven perspective on the problem, facilitating a more effective solution. In healthcare, data analysis of patient demographics and treatment responses can guide personalized therapies.
- Generating Potential Solutions
Identifying potential solutions is a critical step. This often involves brainstorming, considering different perspectives, and exploring alternative approaches. In business, this stage involves analyzing market trends, considering competitors' actions, and evaluating internal resources. This creative stage benefits from the data-driven analysis performed earlier.
- Evaluating and Selecting the Optimal Solution
Evaluating the potential solutions against predetermined criteria is vital. Factors like feasibility, cost-effectiveness, and potential impact are considered. A structured evaluation using metrics and quantitative analysis helps narrow down choices. This process prioritizes solutions that best address the problem, considering resources and constraints.
In conclusion, problem-solving within the "eorme" framework is a structured process that leverages the power of data analysis and rigorous evaluation. This structured approach leads to more informed decisions, greater efficiency, and improved outcomes. By carefully defining problems, collecting and analyzing data, generating potential solutions, and evaluating them, organizations can effectively address challenges and achieve their objectives. This data-driven approach ensures solutions are not based on intuition or conjecture but on evidence, increasing their likelihood of success.
6. Decision Support
Decision support, a critical application of the "eorme" framework, utilizes data-driven insights to inform choices and strategies. The strength of decision support lies in its ability to provide a structured approach to complex situations, reducing reliance on intuition and maximizing the likelihood of optimal outcomes. This structured methodology is particularly valuable in situations where multiple factors influence outcomes and decisions must be evidence-based.
- Data-Informed Options
Decision support systems, enabled by the "eorme" process, offer a range of options based on analyzed data. For example, in financial forecasting, evaluating various market trends and historical data can facilitate informed investment decisions. In healthcare, patient data analysis allows for the identification of optimal treatment plans. These options aren't arbitrary; they are derived from a comprehensive understanding of the problem space, enabling choices that are grounded in evidence and not just conjecture.
- Quantifiable Metrics for Assessment
Decision support often utilizes quantifiable metrics to assess potential outcomes. These metrics are derived from statistical analysis within the "eorme" framework. For example, a business evaluating marketing campaigns can use metrics like conversion rates, customer acquisition costs, and return on investment. The quantifiable nature of these assessments provides a standardized and objective evaluation framework, leading to more rational and reliable decisions.
- Reduced Uncertainty through Forecasting
Predictive modeling, a key component of "eorme," significantly reduces uncertainty in decision-making. By leveraging historical data and statistical analysis, models project future outcomes, enabling anticipatory actions and contingency planning. In supply chain management, forecasting demand allows for proactive inventory adjustments and resource allocation, reducing the risk of stockouts or overstocking. These predictive capabilities are vital for navigating complex and volatile environments, increasing the reliability of decision-making.
- Improved Resource Allocation Based on Insights
The "eorme" framework fosters optimized resource allocation through informed decisions. By analyzing data and projecting outcomes, organizations can strategically allocate resources to maximize return. For example, in manufacturing, analyzing production data and sales forecasts can determine the optimal level of staffing and equipment, streamlining operations and minimizing costs. Understanding the relationships between various factors allows for efficient utilization of available resources.
In conclusion, decision support, as an application of the "eorme" framework, offers a structured and data-driven approach to making choices. Utilizing analyzed data, quantifiable metrics, and predictive models leads to more informed and reliable decisions. This systematic approach allows organizations to navigate complex issues, optimize resource utilization, and increase the likelihood of positive outcomes. The effectiveness of decision support hinges on the reliability and comprehensiveness of the data and the models built within the "eorme" process.
7. System Understanding
System understanding, a critical component of the "eorme" framework, entails a deep comprehension of the intricate relationships and interactions within a complex system. This understanding is not merely descriptive but also predictive, enabling anticipation of system behavior under various conditions. It is the bedrock upon which effective problem-solving and decision-making are built within "eorme." Failure to grasp the system's intricacies can lead to ineffective solutions and unintended consequences. For example, a financial institution neglecting the interconnectedness of various market segments might implement a strategy that benefits one area while jeopardizing another. Likewise, in environmental management, a lack of understanding of complex ecological interactions could have disastrous outcomes.
A comprehensive system understanding within the "eorme" framework fosters the development of more effective models. By acknowledging the interdependence of elements, models gain predictive power beyond the simple extrapolation of trends. For instance, a study of a supply chain using "eorme" would not only analyze individual components but also examine how changes in one partlike a suppliers production capacitymight cascade through the entire system. This holistic view allows for the development of robust models capable of accurately predicting outcomes under a wider range of conditions, including unforeseen disturbances. Understanding the interplay between market demand, production capacity, and transportation logistics allows for more accurate forecasts and proactive adjustments in the supply chain. A deep comprehension of the feedback loops and interactions between variables is key to anticipating and preventing potential disruptions.
Ultimately, system understanding within the "eorme" process ensures that interventions are not merely reactive but are thoughtfully considered actions that address the root causes of issues within the broader system. This proactiveness minimizes unintended consequences and maximizes the likelihood of achieving desired outcomes. While achieving complete system understanding is often challenging due to the complexity of most real-world systems, the "eorme" approach provides a systematic framework for approaching this challenge. Striving for a holistic view of the system, rather than isolated aspects, is crucial to achieving the full potential of the "eorme" process and avoiding the pitfalls of simplified or incomplete models. This deeper understanding drives more robust and effective outcomes across numerous domains, from healthcare to economics.
Frequently Asked Questions about "eorme"
This section addresses common inquiries regarding the "eorme" framework. The following questions and answers aim to clarify key concepts and applications of this analytical approach.
Question 1: What is the core principle behind "eorme"?
The core principle of "eorme" is a structured, data-driven approach to understanding complex systems. It emphasizes a systematic process of data collection, pattern recognition, statistical analysis, predictive modeling, and ultimately, informed decision-making. This framework prioritizes evidence-based solutions rather than relying on assumptions or intuitive judgments. The methodology is designed to uncover underlying relationships and trends that might not be apparent through casual observation.
Question 2: What distinguishes "eorme" from other analytical methods?
"Eorme" distinguishes itself by its comprehensive, multi-faceted approach. Unlike methods focused solely on statistical modeling or data visualization, "eorme" incorporates rigorous steps for data collection quality, pattern recognition across various layers of data, and robust statistical analysis. Crucially, it culminates in predictive modeling, leading to more proactive and anticipatory problem-solving strategies.
Question 3: How does "eorme" apply to various fields?
The "eorme" framework is applicable across numerous fields. Its structured methodology proves valuable in diverse domains such as financial forecasting, supply chain optimization, healthcare diagnostics, environmental modeling, and more. The adaptability of the framework lies in its ability to dissect complex systems, irrespective of their particular characteristics or intricacies.
Question 4: What are the potential limitations of the "eorme" framework?
While "eorme" offers a powerful methodology, limitations exist. The accuracy of predictions depends entirely on the quality and completeness of the data. Complex systems can be inherently unpredictable, and models are only as good as the data they're trained on. Furthermore, interpreting results from intricate analyses demands skilled expertise and robust tools.
Question 5: How can one implement the "eorme" framework in practice?
Implementation requires careful planning and a multidisciplinary approach. Initial steps should involve meticulous data collection and identification of the precise problem. Following this, pattern recognition and statistical analysis are fundamental to developing predictive models. The iterative refinement of these models against independent data sets ensures accuracy and applicability to real-world problems.
In conclusion, "eorme" presents a robust framework for addressing complex issues with a structured, data-driven approach. Understanding its core principles, potential applications, and limitations is crucial for effective implementation and reliable outcomes.
The next section will delve into specific case studies illustrating "eorme" in action across various industries.
Conclusion
The "eorme" framework presents a structured and data-driven approach to addressing complex issues across diverse domains. Key components, including meticulous data collection, sophisticated pattern recognition, robust statistical analysis, predictive modeling, and a thorough understanding of system dynamics, combine to yield insightful solutions. This process, by emphasizing evidence-based methodologies, reduces reliance on intuition and increases the likelihood of successful outcomes. The framework's iterative nature allows for continuous refinement and adaptation to evolving circumstances, ensuring its relevance in navigating intricate and dynamic systems. The practical applications are diverse, encompassing areas from financial forecasting and supply chain optimization to healthcare diagnostics and environmental modeling.
The efficacy of the "eorme" approach hinges on the quality of data utilized and the expertise applied in its analysis. While the framework provides a robust methodology, the challenge lies in understanding and applying the process effectively. Further development of the "eorme" framework should consider advancing predictive models to encompass greater complexity and uncertainty. The evolution of computational resources and data-handling capabilities warrants investigation into how "eorme" can leverage these advances to solve even more intricate problems. Ultimately, the "eorme" framework represents a significant advancement in problem-solving methodologies, offering a valuable tool for informed decision-making in an increasingly complex world. Continued exploration and application of this framework promise to yield significant benefits in a wide array of fields.
You Might Also Like
Heidi Van Pelt: Actress & Model - Latest NewsIsauro Aguirre Execution: Latest Updates & Details
Jeanette Adair Bradshaw: Life & Legacy
How Tall Is Gabriel Macht? Height Revealed!
Anthony Fantano's Wife: Everything You Need To Know
Article Recommendations
- Exploring Mydesi Your Ultimate Guide To Indian Culture And Lifestyle
- Deon Coles Life Partner Insights And Perspectives
- Mark Hamill Net Worth Financial Journey And Success

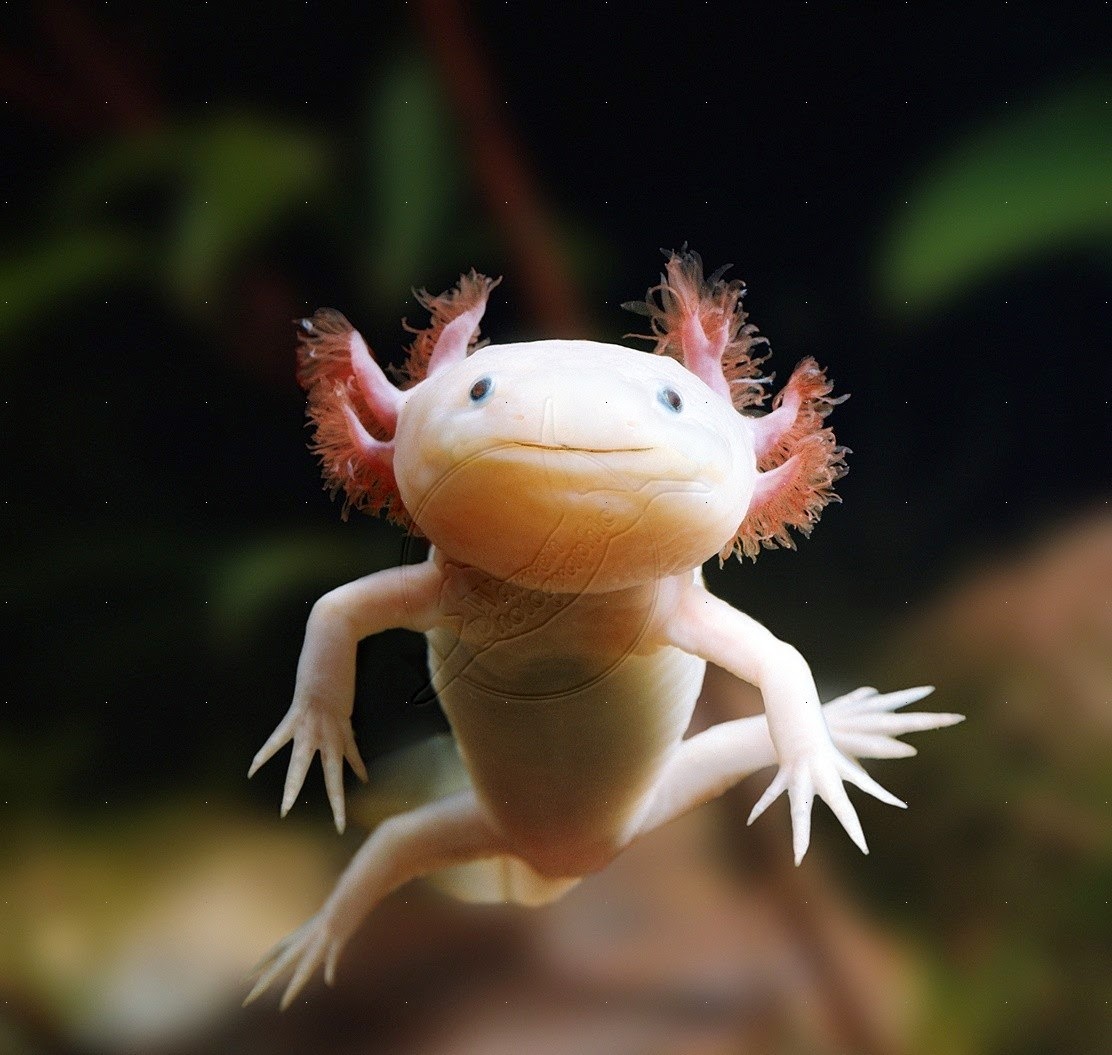